The Journal of Clinical and Preventive Cardiology has moved to a new website. You are currently visiting the old
website of the journal. To access the latest content, please visit www.jcpconline.org.
Basic Research for Clinicians
P Value, Statistical Significance and Clinical Significance
Volume 2, Oct 2013
Padam Singh, PhD Gurgaon, India
J Clin Prev Cardiol. 2013;2(4):202-4
Statistical Test of Significance
The theory of statistical test of significance essentially involves setting of a null Hypothesis and competing alternative hypothesis. The null hypothesis (H0) is a hypothesis of “no difference, no effect, no correlation, no association etc.” The null hypothesis is generally the negation of the research hypothesis.
The alternative hypothesis (H1) is the competing alternative to the null hypothesis and is usually the research hypothesis set out to investigate.
As an example, if we are investigating a new drug for its superiority over a standard drug, the null and alternative hypothesis would be as under:
Null hypothesis (H0): There is no difference in the treatment outcome between the new drug and the standard drug.
The alternative hypothesis (H1): The treatment outcome with the new drug would be better as compared to the standard drug.
Mathematically,
H0: pn = ps,
H1: pn > ps
Where
pn= Efficacy of new drug
ps= Efficacy of standard drug
While drawing an inference in the testing of hypothesis following are used:
- The level of significance (a): The probability of Type I error (rejecting the null hypothesis (H0) when it is true)
- The estimated p-value for given data set of the study.
If calculated p-value is less than the chosen level of significance then the null hypothesis is rejected.
The p value is calculated based on the Null distribution, which is a theatrical distribution of the test statistic when the null hypothesis is true. The commonly used null distributions and their applications are given as under:

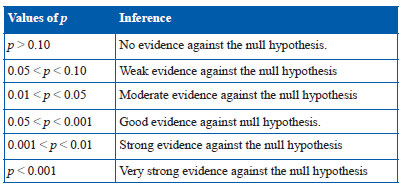
The p-value and null distribution
The p value is calculated based on the Null distribution, which is a theatrical distribution of the test statistic when the null hypothesis is true. The commonly used null distributions and their applications are given as under:

Given the value of the test statistic and the null distribution of the test statistic, it is important to see whether the test statistic is in the middle of the distribution or out in a tail of the distribution. If in the middle of it is consistent with the null hypothesis and out in the tail making the alternative hypothesis seems more plausible. The large values of the test statistic seem more plausible under the alternative hypothesis than under the null hypothesis. The larger values of test statistic corresponds to the smaller the p-value, which are indicative of the stronger evidence against the null hypothesis in favor of the alternative.
Interpretation of p-value
The p-value indicates how probable the results are due to chance.
p=0.05 means that there is a 5% probability that the results are due to random chance.
p=0.001 means that the chances are only 1 in a thousand.
The choice of significance level at which you reject null hypothesis is arbitrary. Conventionally, 5%, 1% and 0.1% levels are used. In some rare situations, 10% level of significance is also used.
Statistical inferences indicating the strength of the evidence corresponding to different values of p are explained as under:
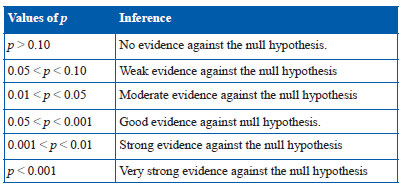
Conventionally, p < 0.05 is referred as statistically significant and p < 0.001 as statistically highly significant. When presenting p values it is a common practice to use the asterisk rating system.
Important in medical research, p values less than 0.05 are often reported as statistically significant as we want there to be only a 5% or less probability that the treatment results, risk factor, or diagnostic results could be due to chance alone. Results that do not meet this threshold are generally interpreted as negative.
p-value and sample size
The magnitude of p-value depends on the sample size. In view of this, following is important to note:
- Given a sufficiently large sample size, even an extremely small difference could be detected as significant. However, the difference may not be of clinical importance.
- Conversely, potentially medically important differences observed in small studies, for which the p values are more than 0.05, are ignored.
- All statistically significant findings at (p < 0.05) are assumed to result from real treatment effects, whereas by definition an average of one in 20 comparisons in which null hypothesis is true will result in p < 0.05.
Clinical Significance
One should keep in mind the difference between statistical significance and clinical significance. The results of a study can be statistically significant but still be too small to be of any practical value.
We might encounter a situation where new treatment shows reduction in pain as statistically significant with a p value of 0.0001. The extremely low p value in this situation indicates that we are really sure that the results are not accidental-- the improvement is really due to the treatment and not just chance. However, it is important to note the magnitude of improvement while interpreting it clinically.
Usually, the extremes are easy to recognize and agree upon. If with the new treatment being investigated, patients get 90% pain relief, we can all agree that this is an effective, worthwhile treatment. But if reduction in pain is just 3%, such a paltry effect may not be of any importance.
It is thus important to decide whether the observed treatment effect is large enough to make a difference.
But what would constitute the smallest amount of improvement (say δ) that would be considered worthwhile. After all we want the new treatment to make a difference. This is tricky. The researchers doing the study should explicitly state what this minimal amount of clinically important improvement is.
One can find the absolute amount of improvement in critical outcome measure, to decide if the improvement looked very large and can cross reference it with other sources to decide whether it met the Medically Important Clinical Difference (MICD). The null and alternative hypothesis could be appropriately framed to detect the Medically Important Clinical Difference as significant.
The Null and Alternative Hypothesis in this situation would be as under:
H0: pn = ps+δ
H1: pn > ps+δ
It is therefore advised, not to just look at the p value but also try to examine if the results are robust enough to be clinically significant with desired improvements (MICD).
References
- Krikwood BR, Sterne JAC. Medical statistics, 2nd edition, Oxford Blackwell Science Ltd; 2003
- Why Publish with JCPC?
- Instructions to the Authors
- Submit Manuscript
- Advertise with Us
- Journal Scientific Committee
- Editorial Policy
Print: ISSN: 2250-3528
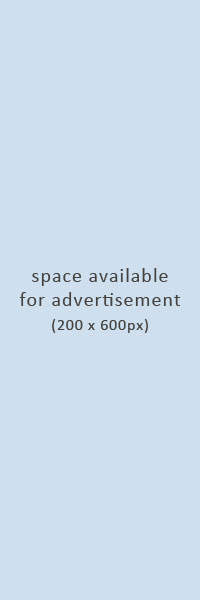